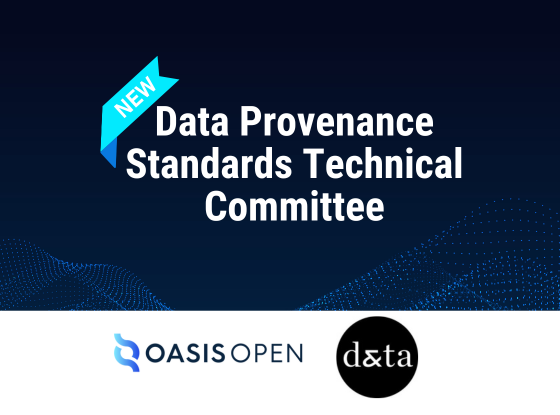
Call for Participation: Data Provenance Standards Technical Committee (DPS TC)
New TC aims to implement consistent tagging and metadata frameworks across data ecosystems—down to database, table, and column levels—to provide comprehensive data lineage and collection details tracking and support responsible data use, privacy, and compliance across all industries.
A new OASIS TC is being formed. The Data Provenance Standards (DPS) TC has been proposed by Cisco, the Data & Trust Alliance, IBM, Intel, Microsoft, Red Hat, and others listed in the charter below. The public TC homepage is here.
All interested parties are welcome to join this TC. The eligibility requirements for becoming a participant in the TC at the first meeting are:
- You must be an employee or designee of an OASIS member organization or an individual member of OASIS, and
- You must join the TC, which members may do by using the Roster “join group” link on the TC’s web page or by clicking here.
To be considered a voting member at the first meeting:
- You must join the TC at least 7 days prior to the first meeting on or before April 1, 2025; and
- You must attend the first meeting of the TC on April 8, 2025. Note: no work, including technical discussions or contributions, may occur prior to the first TC meeting.
Participants also may join the TC at a later time.
If your employer is already on the OASIS TC member roster, you may participate in DPS TC (or any of our TCs) at no additional cost. Find out how.
If your employer is not a member, we’re happy to help you join OASIS. Contact us to discuss your options for TC membership.
Please feel free to forward this announcement to any other appropriate lists. OASIS is an open standards organization; we encourage and welcome your participation.
OASIS Data Provenance Standard Technical Committee Charter
The charter for this TC is as follows:
Section 1: TC Charter |
1.a. TC Name Data Provenance Standards Technical Committee (DPS TC) |
1.b. Statement of Purpose Provenance matters. We understand the sources of food, water, medicine, and capital-essential in our society to gauge quality and trust-and must now work to understand data, the fuel of our increasingly knowledge- and AI-centric world. For the purposes of this document and related TC efforts, provenance, pedigree, and lineage are recognized as distinct but interconnected concepts. The TC will prioritize early efforts to define how these terms-ranging from origin and history to granularity at the geographic, organizational, and individual levels-are scoped and applied to benefit all stakeholders. This will ensure comprehensive, practical, and actionable standards while mitigating ambiguity and scope constraints. Of course, building trust in data starts with transparency of provenance-assessing where data comes from, how it’s created, and whether it can be used legally. Yet, the ecosystem still needs a common language to provide that transparency. Establishing shared provenance standards is foundational to fostering trust in data and AI-driven systems. Over the past 18 months, the Data & Trust Alliance, in collaboration with industry organizations such as the EDM Council and AI Alliance, has worked to normalize and map its data provenance standards to existing initiatives while identifying practical adoption paths. For example, based on recommendations from the AI Alliance, the Data & Trust Alliance’s metadata framework has been integrated into Hugging Face model cards to promote provenance transparency in AI development. Using Version 1.0.0 of the Data Provenance Standards, defined by a working group of industry leaders from the Data & Trust Alliance, the OASIS Data Provenance Standards Technical Committee aims to advance data transparency, accountability, and trust by solidifying provenance standards into a universal data governance norm. This initiative will focus on implementing consistent tagging and metadata frameworks across data ecosystems-down to database, table, and column levels-to provide comprehensive data lineage and collection details tracking and support responsible data use, privacy, and compliance across all industries. The Committee will consider trust in data, ensuring that provenance, lineage, pedigree, and ultimately transparency support trust-building efforts in AI and data ecosystems. The Committee will consider existing trust models where relevant, ensuring alignment with industry best practices while remaining focused on provenance as a key enabler of trust. By establishing these standards, the Committee will enhance data life-cycle management, facilitate regulatory adherence, and reinforce trust in AI-driven and data-dependent applications. The Committee will also explore opportunities for integrating automated tools to generate and validate metadata, ensuring scalability and ease of adoption while maintaining trust and compliance. The goal is to create actionable standards that deliver measurable business value, such as enhanced operational efficiency and trust in AI systems, and to encourage adoption by demonstrating clear ROI for both data providers and consumers. |
1.c. Business Benefits It is expected that these standards will benefit all data and AI stakeholders, including: -data suppliers (e.g., data producers, technology companies)-who will be able to deliver clear and consistent data lineage information, making their datasets more valuable and trustworthy. Compliance can combat piracy and misuse. -data acquirers (e.g., data-driven organizations, regulatory bodies)-who will benefit from greater transparency and being better able to assess the reliability and intended usage of datasets and to request changes or reject data sets when necessary. Higher performing AI tools can be a direct outcome. -end-users (consumers)-who will gain insight into how their data is managed and protected, and thus become more trusting in representative/non-biased data-driven solutions. These standards will: -enable data suppliers to provide standardized, consistent metadata on data lineage and provenance -support data acquirers in managing compliance and mitigating risks associated with data privacy, security, and intellectual property rights -help end-users by ensuring transparency in data handling and increasing trust in digital services. The standards will be relevant to professionals across various domains, including: -data governance professionals (including legal and compliance stewards) -IT and compliance officers -AI and data scientists -business and industry professionals who rely on trusted data for decision-making. Adoption will be driven by enterprise demand for metadata-tagged datasets that offer faster access, reduced compliance risks, and improved decision-making. The availability of automated tools for metadata tagging and validation will significantly lower adoption barriers and costs for data providers. |
1.d. Scope The TC will develop cross-industry standards for defining data provenance, pedigree, lineage, and metadata-tagging frameworks. These will support tags at the database, table, and column levels, as well as metadata for graph databases, NoSQL databases, and data exchanged via APIs and other non-database structures. The scope includes creating guidelines and schemas for managing the data life cycle and tracking provenance, pedigree, and lineage across diverse data architectures and transmission methods. While highly domain-specific adaptations may require additional tailoring by industry groups, these standards are intended to provide a flexible foundation that is applicable across multiple sectors. Additionally, provenance-related geolocation metadata will encompass latitude/longitude, political/geographical boundaries, organizational context, and person-based attributes where relevant, supporting trust assessments based on data origin. The TC will also provide guidance on the development and integration of tools for automating metadata tagging, validation, and transformation, to ensure accuracy and compliance. The scope of these standards does not include tagging for misinformation, disinformation, or malinformation (“mis/dis/mal”); rather, such determinations are beyond provenance and are expected to be derived by users (e.g., AI/ML systems) externally to these specifications. The TC will prioritize datasets that are critical for AI/ML applications and enterprise use cases, balancing comprehensive tagging with practical implementation considerations. |
1.e. Deliverables Expected deliverables include: -Committee specifications for standardized data provenance tags -Committee notes and/or guides on how to implement the standards -supporting documentation such as glossaries, UML models, and metadata requirements documents -guidelines for integrating tools to automate metadata tagging, validation, and life-cycle management -additional deliverables as determined by the Technical Committee, such as reference implementations, case studies, interoperability frameworks, based on ongoing needs and industry developments, or a study on how the standards align and enable compliance (for AI providers) with transparency regulation in the AI space as well as the benefits of the standards to data providers. The Technical Committee aims to release initial drafts by mid-2025. This will be followed by public feedback phases and iterative refinements, with the goal of finalizing and publishing the standards by late 2025. Timelines may be adjusted based on industry input and the progress of Committee discussions. |
1.f. IPR Mode Non-assertion |
1.g. Audience Participants will include AI ethics and privacy specialists, data governance and compliance professionals, IT managers, and regulatory advisors from various industries, particularly finance, healthcare, and retail. |
1.h. Language English |
(Optional References for Section 1) Data & Trust Alliance website detailing work to date on data provenance standards GitHub repository with technical specifications Standards Executive Briefing IBM’s IBV report with results of data provenance standards testing – 58% reduction in data clearance processing time for third-party data and a 62% reduction in data clearance processing time for IBM-owned or generated data Use cases –four key areas of practice to help with understanding and sharing the standards |
Section 2: Additional Information |
2.a. Identification of Similar Work Similar or related work includes: -NIST (https://www.nist.gov/itl/ai-risk-management-framework) -EDM Council (https://edmcouncil.org/frameworks/cdmc/) with which the Data & Trust Alliance has collaborated and mapped to the CDMC; in the upcoming CDMC refresh we will have full alignment in our metadata -MIT Media Lab (https://www.media.mit.edu/projects/data-provenance-for-ai/overview/) with which the Data & Trust Alliance has coordinated and determined that there are synergies but no duplication of effort -W3C (https://www.w3.org/TR/prov-dm/) which is focused on web provenance; the Data & Trust Alliance has mapped its metadata to components of PROV, demonstrating minimal overlap -complementary initiatives including the ISO standards for data management, the FAIR principles, and other industry-specific data governance frameworks – the AI Alliance having adopted the standards for its definition of data trust -OSIM (Open Supplychain Information Modeling (https://www.oasis-open.org/tc-osim/)-the framework for structuring and exchanging supply chain data, enabling interoperability, transparency, and efficiency across industries -DAD-CDM (Common Data Model for Defending Against Deception, https://github.com/DAD-CDM) which provides a standardized data model for AI and data development, thus enhancing interoperability, consistency, and efficiency across diverse data ecosystems -COSAI (https://www.coalitionforsecureai.org/) which is focused on developing and promoting security standards, best practices, and policies to ensure the safe and responsible development and deployment of AI technologies -Apache Atlas (https://atlas.apache.org/) which provides metadata management and governance capabilities that align with the data provenance standards by enabling structured metadata tagging and lineage tracking across enterprise data ecosystems -OpenLineage (https://openlineage.io/) which offers an open framework for capturing and standardizing data lineage, complementing the data provenance standards by ensuring transparency and traceability in data workflows -Community Data License Agreement (CDLA) (https://cdla.dev) which offers collaborative licenses designed to facilitate the open sharing, access, and use of data among individuals and organizations. The DPS TC will differentiate itself by creating a cross-industry standard that focuses on comprehensive data provenance, pedigree, and lineage tracking, responsible (from an IP and privacy perspective) AI use, and regulatory compliance support, filling a gap for generalizable and adaptable provenance standards. |
2.b. First TC Meeting April 8, 2025 @ 1pm ET, via a virtual format |
2.c. Ongoing Meeting Schedule Meetings will be held monthly. |
2.d. TC Proposers Lisa Bobbitt, Cisco, lbobbitt@cisco.com Kristina Podnar, Data & Trust Alliance kpodnar@dataandtrustalliance.org Saira Jesani, Data & Trust Alliance sjesani@dataandtrustalliance.org Asmae Mhassni, Intel, asmae.mhassni@intel.com Kelsey Schulte, Intel, kelsey.schulte@intel.com Mic Bowman, Intel, mic.bowman@intel.com Peter Koen, Microsoft, jaywhite@microsoft.com Babak Jahromi, Microsoft, babakj@microsoft.com Jay White, Microsoft, jaywhite@microsoft.com Stefan Hagen, Individual, stefan@hagen.link Janaye Minter, NSA, vjminte@uwe.nsa.gov Duncan Sparrell, SFractal, duncan@sfractal.com Roman Zhukov, RedHat, rzhukov@redhat.com Lee Cox, IBM, Lee.Cox@uk.ibm.com |
2.e. Primary Representatives’ Support I, Omar Santos, as OASIS primary representative for Cisco, confirm our support for the Data Provenance Standard TC and our participants listed above. I, Kristina Podnar, as OASIS primary representative for Data & Trust Alliance, confirm our support for the Data Provenance Standard TC and our participants listed above. I, Jeffrey Borek, as OASIS primary representative for IBM, confirm our support for the Data Provenance Standard TC and our participants listed above. I, Dhinesh Manoharan, as OASIS primary representative for Intel, confirm our support for the Data Provenance Standard TC and our participants listed above. I, Jay White, as OASIS primary representative for Microsoft, confirm our support for the Data Provenance Standard TC and our participants listed above. I, Vincent Boyle, as OASIS primary representative for National Security Agency, confirm our support for the Data Provenance Standard TC and our participants listed above. I, Mark Little, as OASIS primary representative for RedHat, confirm our support for the Data Provenance Standard TC and our participants listed above. |
2.f. TC Convener Kristina Podnar, Data & Trust Alliance, kpodnar@dataandtrustalliance.org |
2.g. Anticipated Contributions Standards Executive Briefing GitHub repository with technical data provenance standards specifications, code snippets, documentation for standards adoption Use cases – four key areas of practice to help with understanding and sharing the standards Metadata generator – the TC will assess the feasibility of existing prototypes, such as the metadata generator, and recommend enhancements to align with the standards The standards may serve as a precursor to broader frameworks like AI Bills of Materials (AI BOMs), enhancing traceability and compliance. |
2.h. FAQ Document https://dataandtrustalliance.org/work/data-provenance-standards |
2.i. Work Product Titles and Acronyms Data Provenance Metadata Specification Data Lineage Standard for AI Compliance Data Transparency and Accountability Standards |